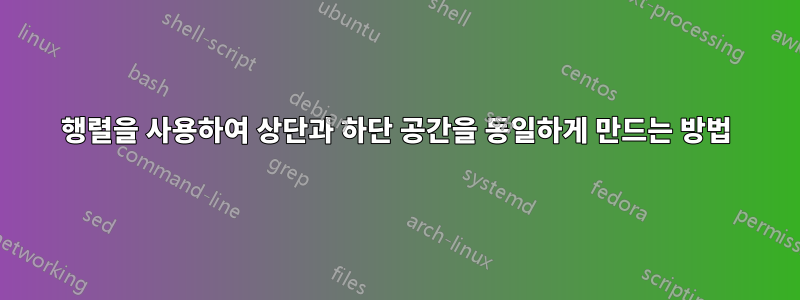
나는 다음을 시도했다:
\documentclass{article}
\usepackage{amsmath}%amssymb,
\usepackage{bm}
\begin{document}
Outputs to machine learning models are also often represented as vectors. For instance, consider an object recognition model that takes an image as input and emits a set of numbers indicating the probabilities that the image contains a dog, human, or cat, respectively. The output of such a model is a three element vector $\vec{y} = \begin{bmatrix}y_{0}\\y_{1}\\y_{2}\\\dfrac{1}{2}\end{bmatrix}$, where the number $y_{0}$ denotes the probability that the image contains a dog, $y_{1}$ denotes the~probability that the image contains a human, and $y_{2}$ denotes the probability that the image contains a cat. Figure~\ref{fig:vec_out} shows some possible input images and corresponding output vectors.
\begin{align*}
p\left( x \right)
&= \overbrace{ \pi_{1}}^{0.33}\mathcal{N}\left( \vec{x}; \, \overbrace{ \vec{\mu}_{1} }^{\begin{bmatrix}
152\\55
\end{bmatrix}} \overbrace{ \bm{\Sigma}_{1}}^{ \begin{bmatrix}
20 &0\\0 &28
\end{bmatrix} } \right)
+ \overbrace{ \pi_{2} }^{0.33} \mathcal{N}\left(\vec{x}; \, \overbrace{ \vec{\mu}_{2} }^{ \begin{bmatrix}
175\\70
\end{bmatrix} }, \overbrace{ \bm{\Sigma}_{2}}^{ \begin{bmatrix}
35 & 39\\39 & 51
\end{bmatrix} } \right)\\
&+ \overbrace{ \pi_{3} }^{0.33} \mathcal{N}\left(\vec{x}; \, \overbrace{ \vec{\mu}_{3} }^{ \begin{bmatrix}
135\\40
\end{bmatrix} }, \overbrace{ \bm{\Sigma}_{3}}^{ \begin{bmatrix}
10 & 0\\0 & 10
\end{bmatrix} } \right)
\end{align*}
\end{document}
출력은 다음과 같이 생성됩니다.
행렬의 위쪽과 아래쪽에 동일한 공간을 만들려면 어떻게 해야 합니까? 조언해주세요
또한, 왜 이런 일이 일어났는지 설명해 주는 것이 더 도움이 됩니다.
답변1
이것이 원하는 것인지 확실하지 않지만 각 부분의 내용을 \vcenter{\hbox{$ ... $}}
.
\documentclass{article}
\usepackage{amsmath}%amssymb,
\usepackage{bm}
\begin{document}
\begin{align*}
p\left( x \right)
&= \overbrace{ \pi_{1}}^{0.33}\mathcal{N}\left(\vcenter{\hbox{$ \vec{x}; \, \overbrace{ \vec{\mu}_{1} }^{\begin{bmatrix}
152\\55
\end{bmatrix}} \overbrace{ \bm{\Sigma}_{1}}^{ \begin{bmatrix}
20 &0\\0 &28
\end{bmatrix} } $}}\right)
+ \overbrace{ \pi_{2} }^{0.33} \mathcal{N}\left(\vcenter{\hbox{$ \vec{x}; \, \overbrace{ \vec{\mu}_{2} }^{ \begin{bmatrix}
175\\70
\end{bmatrix} }, \overbrace{ \bm{\Sigma}_{2}}^{ \begin{bmatrix}
35 & 39\\39 & 51
\end{bmatrix} } $}}\right)\\
&+ \overbrace{ \pi_{3} }^{0.33} \mathcal{N}\left(\vcenter{\hbox{$ \vec{x}; \, \overbrace{ \vec{\mu}_{3} }^{ \begin{bmatrix}
135\\40
\end{bmatrix} }, \overbrace{ \bm{\Sigma}_{3}}^{ \begin{bmatrix}
10 & 0\\0 & 10
\end{bmatrix} } $}}\right)
\end{align*}
\end{document}
답변2
좋은 답변을 받았지만 다음과 같은 조치를 취하시기를 진심으로 제안합니다 \overbrace
.
\documentclass{article}
\usepackage{amsmath}
\usepackage{bm}
\begin{document}
Outputs to machine learning models are also often represented as vectors.
For instance, consider an object recognition model that takes an image as
input and emits a set of numbers indicating the probabilities that the
image contains a dog, human, or cat, respectively. The output of such
a model is a three element vector
$\vec{y} = [\begin{matrix}y_{0} & y_{1} & y_{2} & \frac{1}{2}\end{matrix}]^T$,
where the number $y_{0}$ denotes the probability that the image contains a dog,
$y_{1}$ denotes the~probability that the image contains a human, and $y_{2}$
denotes the probability that the image contains a cat. Figure~\ref{fig:vec_out}
shows some possible input images and corresponding output vectors.
\begin{gather*}
p(x) = \pi_{1} \mathcal{N} ( \vec{x}; \, \vec{\mu}_{1}, \bm{\Sigma}_{1})
+ \pi_{2} \mathcal{N} ( \vec{x}; \, \vec{\mu}_{2}, \bm{\Sigma}_{2})
+ \pi_{3} \mathcal{N} ( \vec{x}; \, \vec{\mu}_{3}, \bm{\Sigma}_{3})
\\[1ex]
\begin{aligned}
\pi_1&=0.33 & \pi_2&=0.33 & \pi_3&=0.33
\\
\vec{\mu}_{1}&=\begin{bmatrix} 152 \\ 55 \end{bmatrix}, &
\vec{\mu}_{2}&=\begin{bmatrix} 175 \\ 70 \end{bmatrix}, &
\vec{\mu}_{3}&=\begin{bmatrix} 135 \\ 40 \end{bmatrix}
\\
\bm{\Sigma}_{1}&=\begin{bmatrix} 20 & 0 \\ 0 & 28 \end{bmatrix}, &
\bm{\Sigma}_{2}&=\begin{bmatrix} 35 & 39 \\ 39 & 51 \end{bmatrix}, &
\bm{\Sigma}_{3}&=\begin{bmatrix} 10 & 0 \\ 0 & 10 \end{bmatrix}
\end{aligned}
\end{gather*}
\end{document}
행 벡터의 전치로 작성된 열 벡터에 주목하세요. 이는 선 사이의 간격을 방지합니다.
대체 정렬 사용
\documentclass{article}
\usepackage{amsmath}
\usepackage{bm}
\begin{document}
Outputs to machine learning models are also often represented as vectors.
For instance, consider an object recognition model that takes an image as
input and emits a set of numbers indicating the probabilities that the
image contains a dog, human, or cat, respectively. The output of such
a model is a three element vector
$\vec{y} = [\begin{matrix}y_{0} & y_{1} & y_{2} & \frac{1}{2}\end{matrix}]^T$,
where the number $y_{0}$ denotes the probability that the image contains a dog,
$y_{1}$ denotes the~probability that the image contains a human, and $y_{2}$
denotes the probability that the image contains a cat. Figure~\ref{fig:vec_out}
shows some possible input images and corresponding output vectors.
\begin{alignat*}{3}
p(x) = \pi_{1} &\mathcal{N} ( \vec{x}; \, \vec{\mu}_{1}, \bm{\Sigma}_{1})
&{}+ \pi_{2} &\mathcal{N} ( \vec{x}; \, \vec{\mu}_{2}, \bm{\Sigma}_{2})
&{}+ \pi_{3} &\mathcal{N} ( \vec{x}; \, \vec{\mu}_{3}, \bm{\Sigma}_{3})
\\[1ex]
\pi_1&=0.33 & \pi_2&=0.33 & \pi_3&=0.33
\\
\vec{\mu}_{1}&=\begin{bmatrix} 152 \\ 55 \end{bmatrix}, &
\vec{\mu}_{2}&=\begin{bmatrix} 175 \\ 70 \end{bmatrix}, &
\vec{\mu}_{3}&=\begin{bmatrix} 135 \\ 40 \end{bmatrix}
\\
\bm{\Sigma}_{1}&=\begin{bmatrix} 20 & 0 \\ 0 & 28 \end{bmatrix}, &
\bm{\Sigma}_{2}&=\begin{bmatrix} 35 & 39 \\ 39 & 51 \end{bmatrix}, &
\bm{\Sigma}_{3}&=\begin{bmatrix} 10 & 0 \\ 0 & 10 \end{bmatrix}
\end{alignat*}
\end{document}
완성도를 높이기 위해 제안된 작업을 수행하는 방법은 다음과 같습니다. 왜 그것이 정말 나쁜지 보여주기 위해 큰 인라인 열 벡터를 남겨 두었습니다.
출력을 비교하면 의심의 여지가 없습니다.
\documentclass{article}
\usepackage{amsmath}
\usepackage{bm}
\usepackage{delarray}
\begin{document}
Outputs to machine learning models are also often represented as vectors.
For instance, consider an object recognition model that takes an image as
input and emits a set of numbers indicating the probabilities that the
image contains a dog, human, or cat, respectively. The output of such
a model is a three element vector
$\vec{y} = \begin{bmatrix}y_{0} \\ y_{1} \\ y_{2} \\ \dfrac{1}{2}\end{bmatrix}$,
where the number $y_{0}$ denotes the probability that the image contains a dog,
$y_{1}$ denotes the~probability that the image contains a human, and $y_{2}$
denotes the probability that the image contains a cat. Figure~\ref{fig:vec_out}
shows some possible input images and corresponding output vectors.
\begin{equation*}
\begin{aligned}
p(x)
&= \overbrace{ \pi_{1}}^{0.33}\mathcal{N}
\begin{array}[b]({c})
\vec{x}; \, \overbrace{ \vec{\mu}_{1} }^{\begin{bmatrix}
152\\55
\end{bmatrix}} \overbrace{ \bm{\Sigma}_{1}}^{ \begin{bmatrix}
20 &0\\0 &28
\end{bmatrix} }
\end{array}
+ \overbrace{ \pi_{2} }^{0.33} \mathcal{N}
\begin{array}[b]({c})
\vec{x}; \, \overbrace{ \vec{\mu}_{2} }^{ \begin{bmatrix}
175\\70
\end{bmatrix} }, \overbrace{ \bm{\Sigma}_{2}}^{ \begin{bmatrix}
35 & 39\\39 & 51
\end{bmatrix} }
\end{array}\\
&+ \overbrace{ \pi_{3} }^{0.33} \mathcal{N}
\begin{array}[b]({c})\vec{x}; \, \overbrace{ \vec{\mu}_{3} }^{ \begin{bmatrix}
135\\40
\end{bmatrix} }, \overbrace{ \bm{\Sigma}_{3}}^{ \begin{bmatrix}
10 & 0\\0 & 10
\end{bmatrix} }
\end{array}
\end{aligned}
\end{equation*}
\end{document}
답변3
\left( ... \right)
의 세 인스턴스를 로 바꾸는 것으로 충분합니다 \bigl( ... \bigr)
. 더 큰 구조의 두 번째 인수 \overbrace
는 정의보다는 설명이므로 (이제 더 이상 키가 크지 않음) 괄호로 묶을 필요가 없습니다.
\vec{y}
아, 그리고 환경 앞에 있는 단락의 정의에 많은 관심을 끌고 싶지 않다면 align*
열 벡터가 아닌 행 벡터로 작성하겠습니다.
\documentclass{article}
\usepackage{amsmath,amssymb,bm}
\begin{document}
Outputs to machine learning models are also often represented as vectors. For instance, consider an object recognition model that takes an image as input and emits a set of numbers indicating the probabilities that the image contains a dog, human, or cat, respectively. The output of such a model is a three element vector
$\vec{y} = \begin{bmatrix} y_{0} & y_{1} & y_{2} \end{bmatrix}'$,
where the number $y_{0}$ denotes the probability that the image contains a dog, $y_{1}$ denotes the~probability that the image contains a human, and $y_{2}$ denotes the probability that the image contains a cat. Figure~\ref{fig:vec_out} shows some possible input images and corresponding output vectors.
\begin{align*}
p(x)
&=\overbrace{ \pi_{1}\mathstrut}^{0.33}\mathcal{N}
\bigl( \vec{x};
\overbrace{ \vec{\mu}_{1} }^{
\begin{bmatrix} 152\\55 \end{bmatrix}} ,
\overbrace{ \bm{\Sigma}_{1}}^{
\begin{bmatrix} 20 &0\\0 &28 \end{bmatrix} }
\bigr)
+\overbrace{ \pi_{2}\mathstrut}^{0.33} \mathcal{N}
\bigl(\vec{x};
\overbrace{ \vec{\mu}_{2} }^{
\begin{bmatrix} 175\\70 \end{bmatrix} },
\overbrace{ \bm{\Sigma}_{2}}^{
\begin{bmatrix} 35 & 39\\39 & 51 \end{bmatrix} }
\bigr)
\\[2\jot] % insert a bit more vertical whitespace
&\quad+\overbrace{ \pi_{3}\mathstrut}^{0.33} \mathcal{N}
\bigl(\vec{x};
\overbrace{ \vec{\mu}_{3} }^{
\begin{bmatrix} 135\\40 \end{bmatrix} },
\overbrace{ \bm{\Sigma}_{3}}^{
\begin{bmatrix} 10 & 0\\0 & 10 \end{bmatrix} }
\bigr)
\end{align*}
\end{document}